
Empower Your Infrastructure for AI Solution Deployment
AI-customized inferencing card with 12 TPUs designed in Saudi Arabia for delivering high performance use for neural network workload at production phase which takes you to the next step from AI-possible to AI-proven.
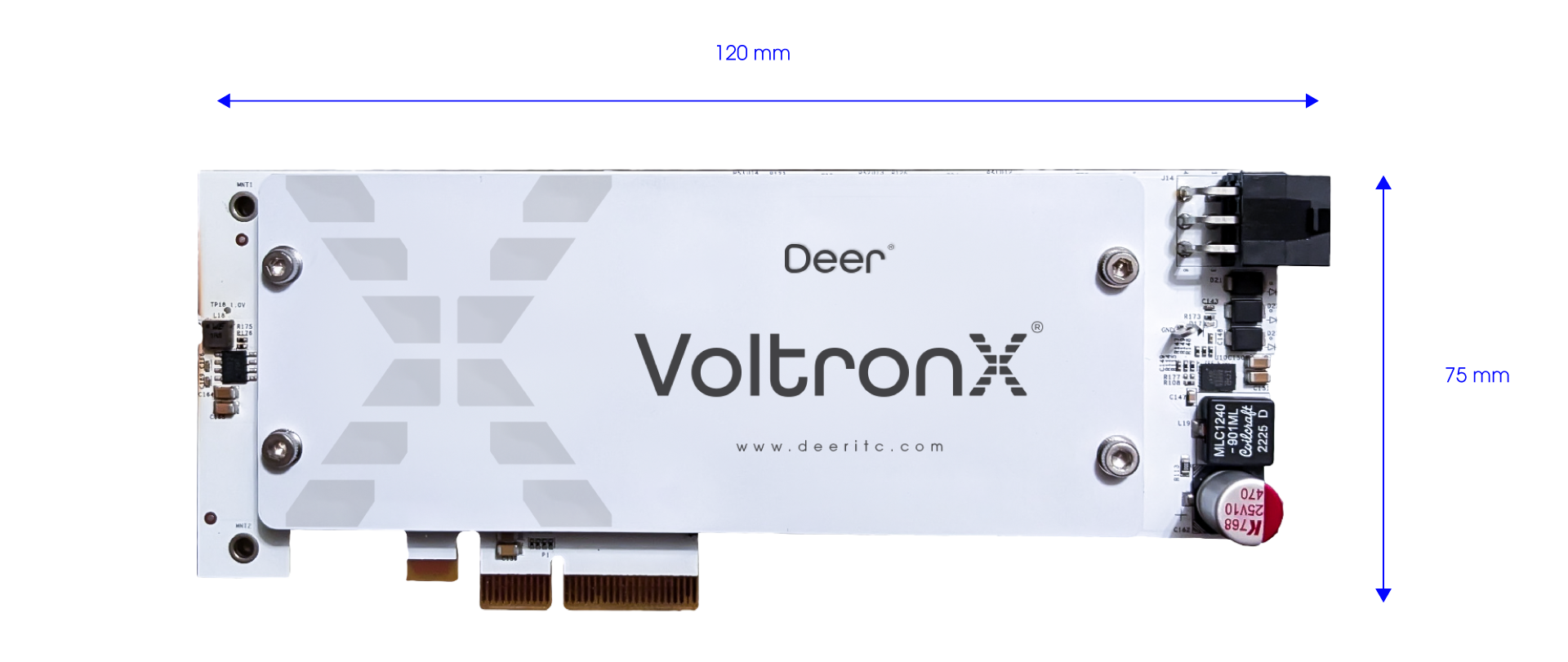
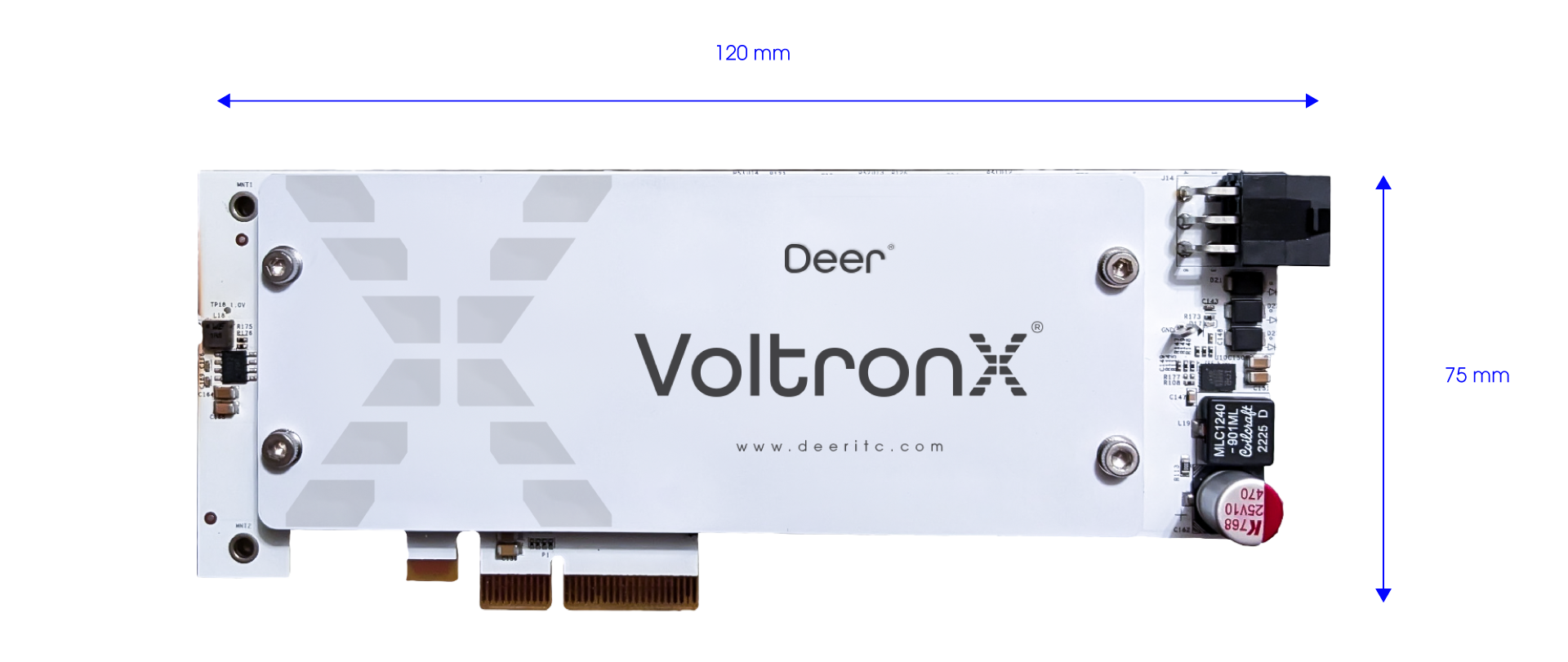
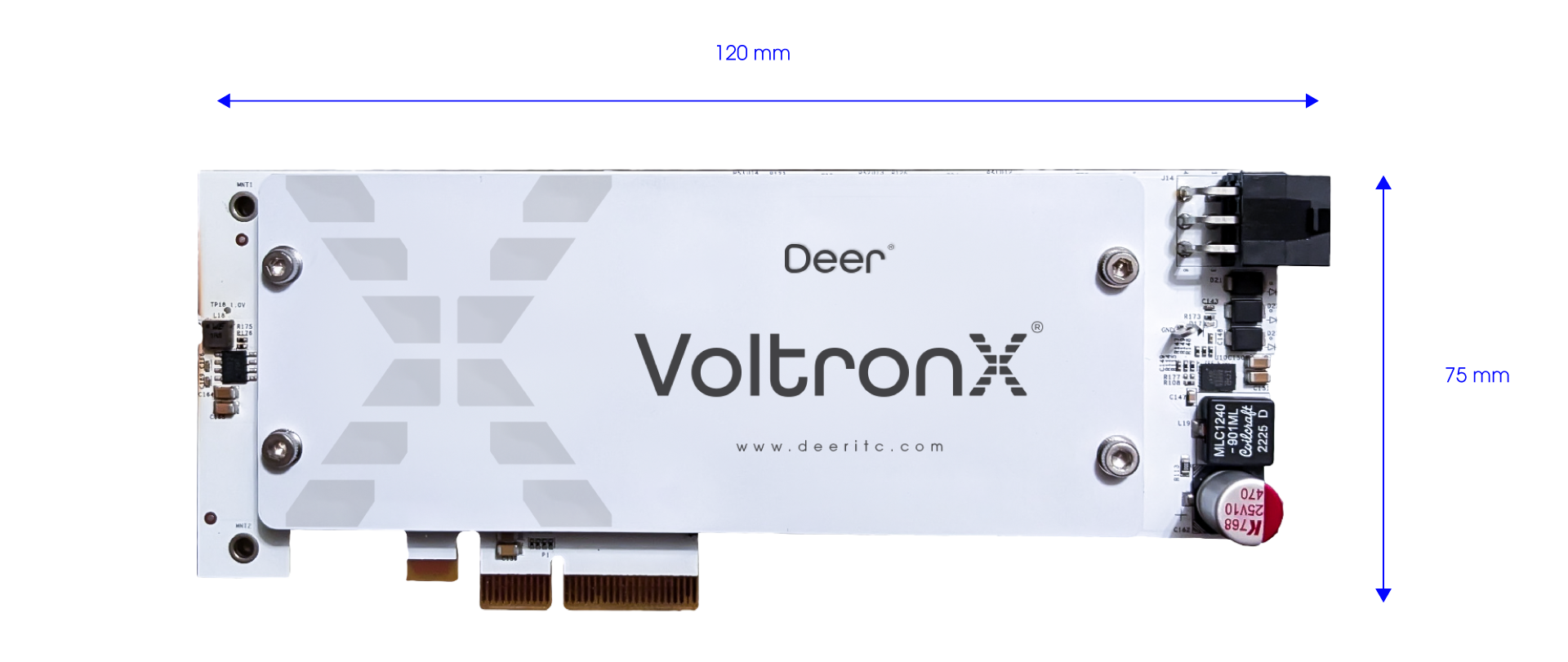
AI-Accelerator PCIe Card
Less Energy, High Efficiency
Deer ITC card
- ASIC design for AI inference acceleration.
- Multiple AI models run in parallel.
- Support up to 48 streams.
- Achieve low latency with AI models pipelining.
- Low power consumption of just 21 Watts.
VoltronX Provides AI Acceleration with
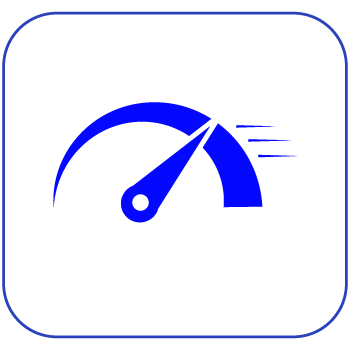
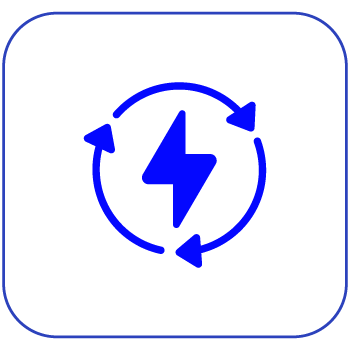
Lower Power Consumption
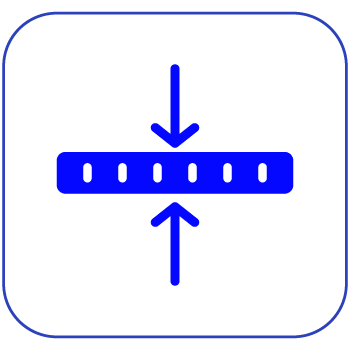
Streamline
Design
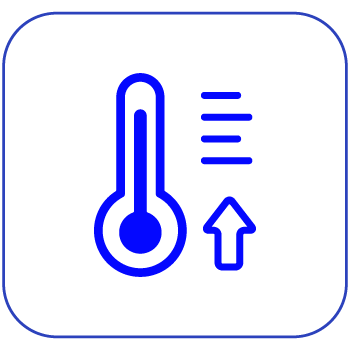
Support Wide Temperature
Simultaneous ML Inferences with Low Latency
Operate multiple AI models in parallel.
In situations where multiple models need to be run, you can assign each model to a dedicated Edge TPU, enabling them to run in parallel, thus achieving peak performance.
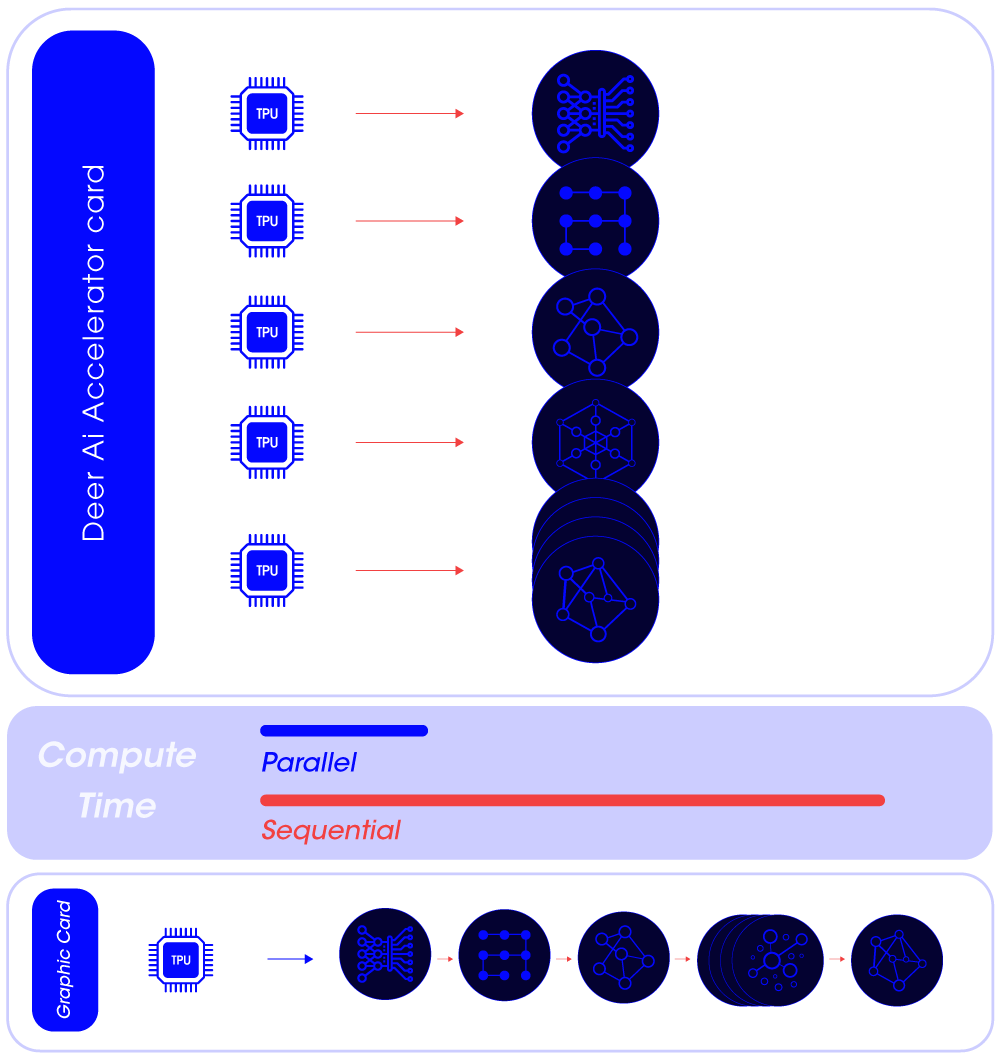
Features
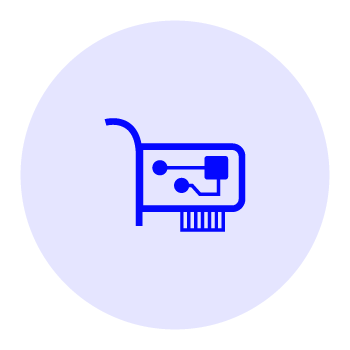
Support TensorFlow Lite machine learning framework
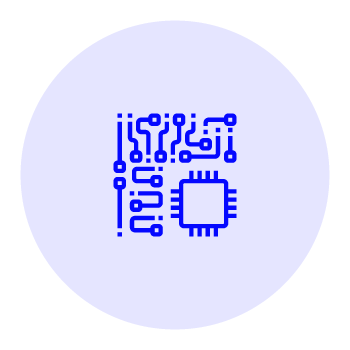
Easy “Plug & Play” installation
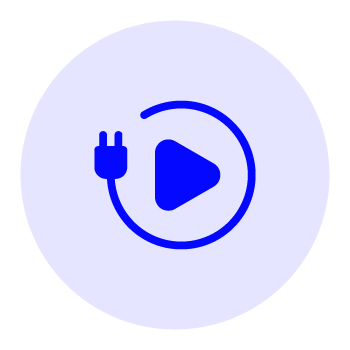
Compliant with any motherboard
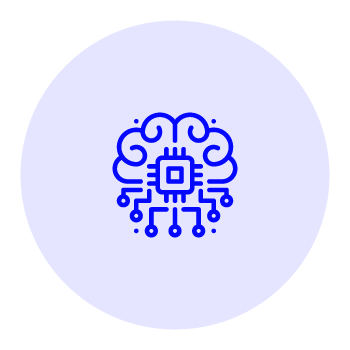
Compliant with PCIe Specification 2.0 x 16 expansion slot
Boosting ML Performance through Model-Pipelining Innovation
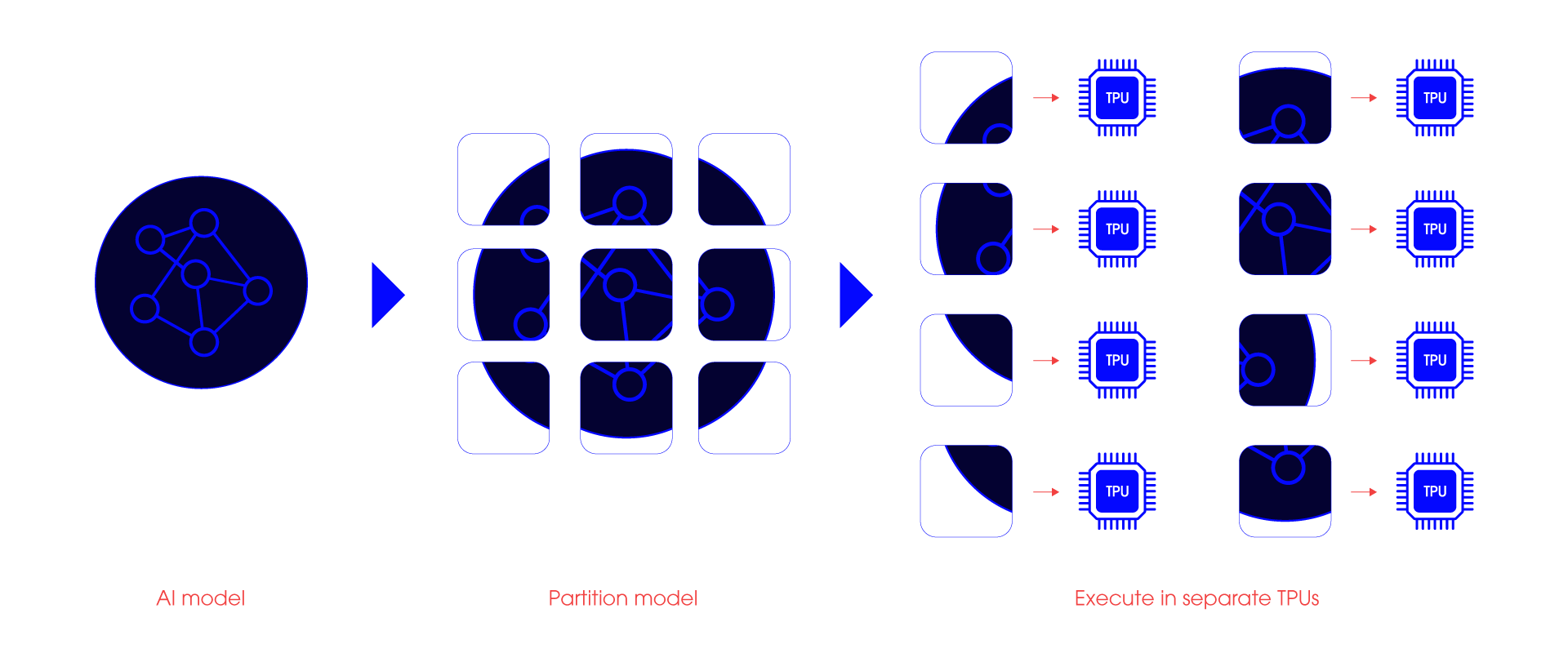
In scenarios demanding rapid responses or the execution of large models, our pipelining technology allows you to break down models into multiple smaller segments.
Segment and Execute: Distribute smaller models across various Edge TPUs.
Swift Responsiveness: Enhance throughput in high-speed applications.
Latency Reduction: Minimize overall latency for large models.
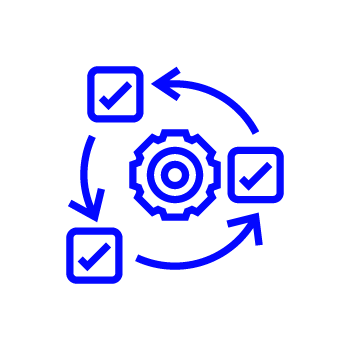
Do more with less energy
Designed with energy efficiency in mind, VoltronX Card is equipped with
excellent thermal stability to achieve inference acceleration with multiple Edge TPUs.
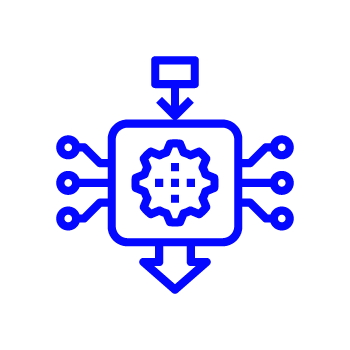
Simultaneous inferencing
Execute your models concurrently on a single or multiple Edge TPU by co-compiling the models so they share the Edge TPU scratchpad memory.
Deploy your AI Model Easily on VoltronX
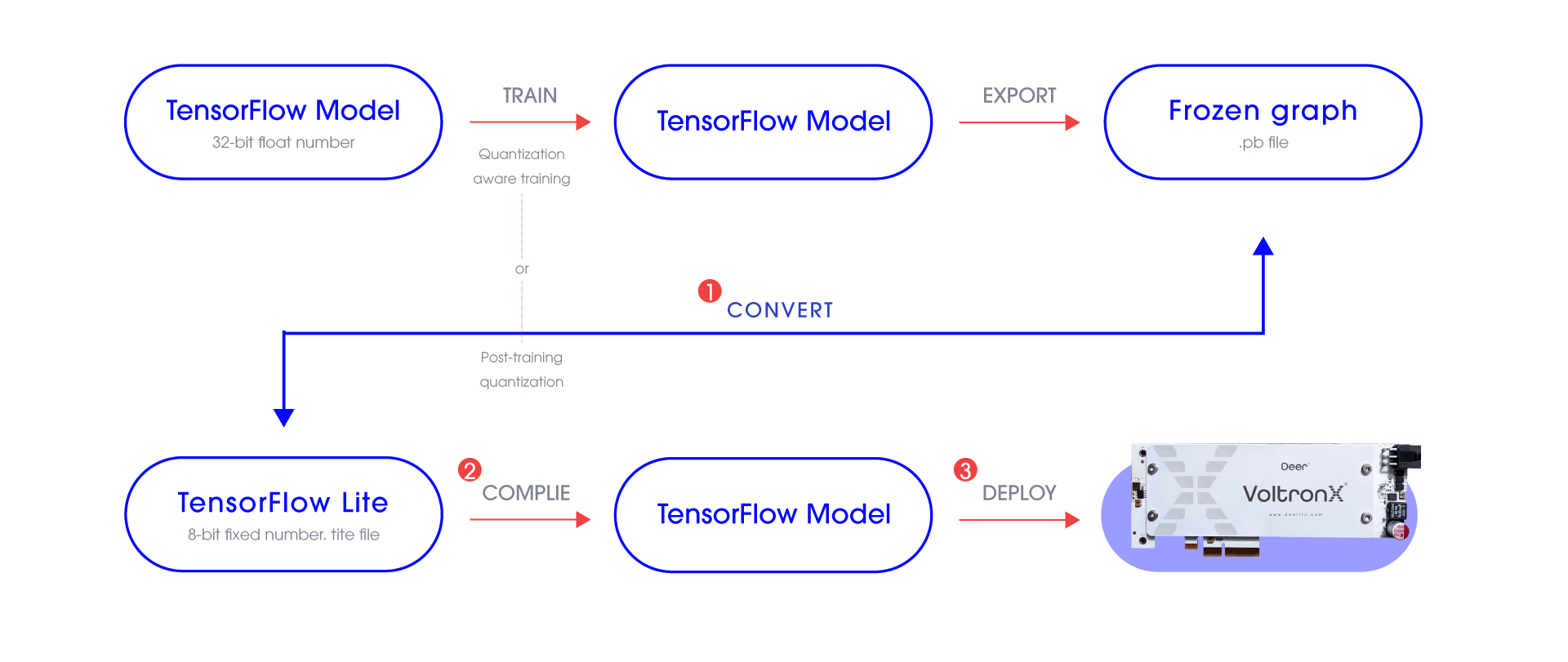
Lite converter : Converts TensorFlow models (with .pb file extension) to TensorFlow Lite models (with .tflite file extension).
Compiler : A command-line tool that compiles a TensorFlow Lite models (with .tflite file extension) into files that can be run on an Edge TPU.
Deploy : To execute AI models via PyCoral API (Python) or Libcoral API (C++).
ML Model Requirements
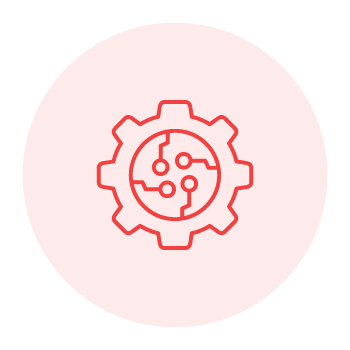
ML Framework Support
TensorFlow Lite
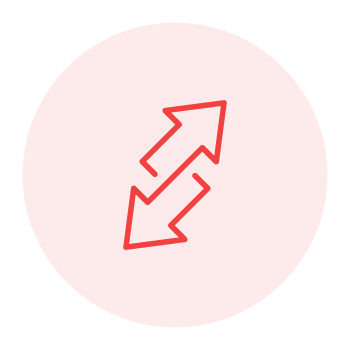
Model Conversion
Tensorflow parameters are Quantizated ( 8 bit fixed-point numbers, int8 )
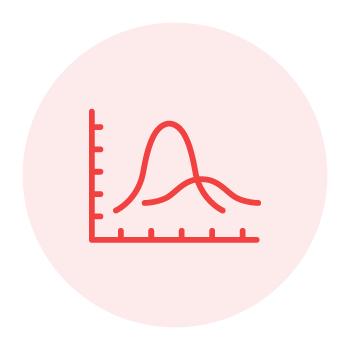
Quantization
TensorFlow model to TensorFlow lite model via TensorFlow converter tool
On-Device Intelligence for a Wide Range of Applications
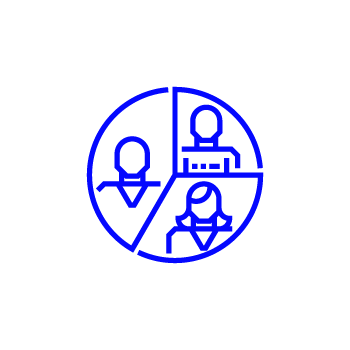
Image segmentation
Identifies various objects in an image and their location on a pixel-by-pixel basis.
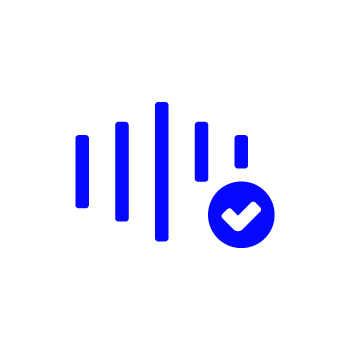
Key-phrase detection
Listens to audio samples and quickly recognizes known words and phrases
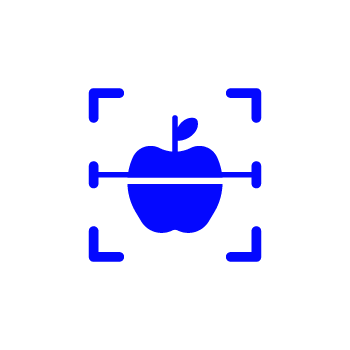
Object detection
Draws a square around the location of various recognized objects in an image
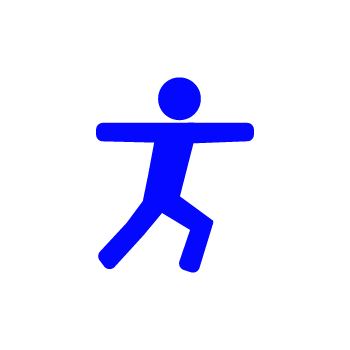
Pose estimation
Estimates the poses of people in an image by identifying various body joints
Technical Specifications
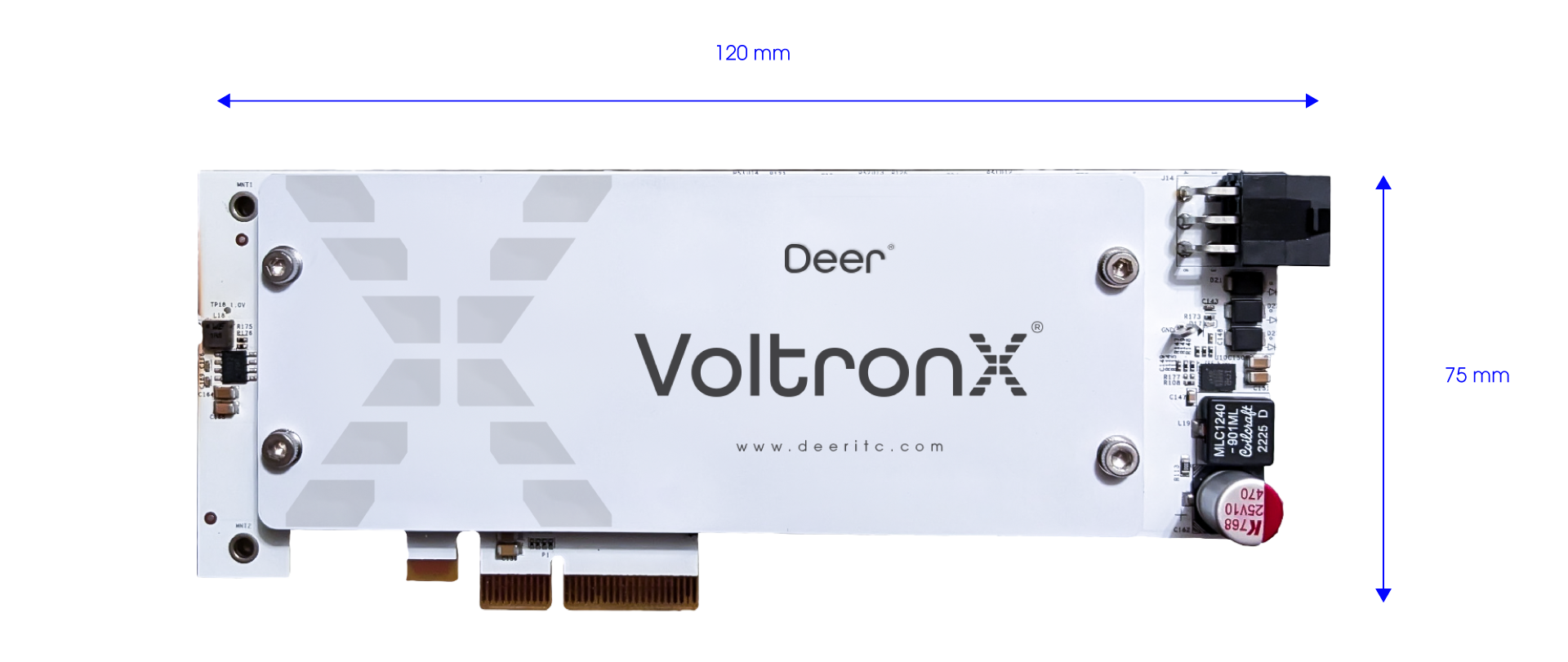
Specifications
Main Chip | Core | Google® Coral Edge TPU Processor |
Interface | Technology | PCI Express 2.0 x16 |
Supported Framework | TensorFlow Lite | |
Software | Precision | INT8 |
Performance | 48 TOPS | |
Thermal Solution | ||
Power | Power Consumption | 24 W |
Operating System | Windows, Linux, OSX | Verified by Google |
Operating Temperature | 0~55°C | |
Environment | Non-Operating Temperature | -40~85°C |
Relative Humidity | 0%~85% | |
Width | 8.1mm | |
Dimension | Height | 126.3mm |
Depth | 186.3mm | |
Weight | Weight | 203 g |
Experience AI Cutting-Edge Solutions Now
Copyright @ 2024. All rights reserved for Deer